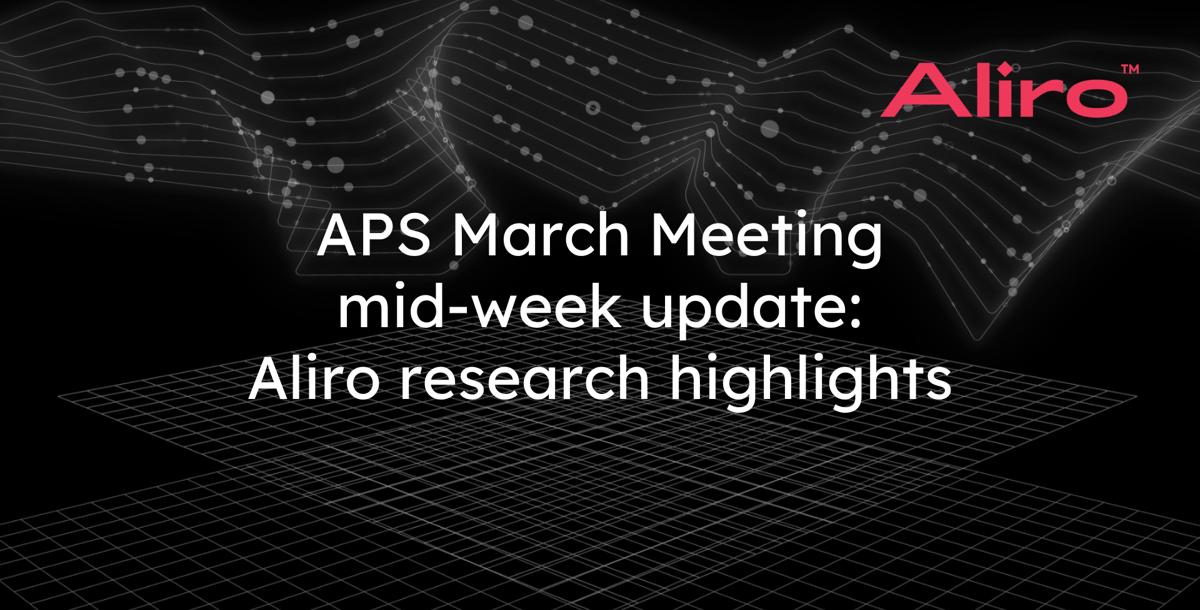
If you’re like us, you’ve been busy jumping between APS March Meeting talks nonstop the last couple days. While we’re missing the hallway run-ins and other unique parts of an in-person conference, this year’s virtual APS has still provided hours of interesting research. To help with your watching list for the second half of the conference, we’ve put together a preview of some talks from Aliro researchers on quantum computing and quantum communication. If you find any of these descriptions interesting, be sure to check out the talks to hear the full story!
Toffoli Gate Depth Reduction in Fixed Frequency Transmon Qutrits
When: Wednesday, March 17 | 4PM EDT (Session P32)
Presenter: Alexey Galda (James Franck Institute, University of Chicago)
Link: here [Registration required]
Quantum computation is conventionally performed using binary quantum logic by operating on quantum bits, or qubits. However, most quantum devices naturally have multiple accessible energy levels beyond the lowest two traditionally used to define a qubit. While unintentional occupation of these higher energy states introduces computational errors, intentional use of these states can provide benefits that are potentially useful in the noisy quantum compute era. We present how, using Qiskit Pulse, one can program a quantum computer over the cloud at the pulse level and implement ternary logic operations on fixed-frequency transmons. From this, we realize a three-qubit Toffoli gate using two different decompositions with 50% fewer (3 instead of 6) two-transmon gates, when compared with the usual binary implementation using CNOT gates.We discuss the theoretical requirements for such qutrit decompositions and our experimental progress of implementing them on pulse-control-enabled devices made available by IBM Quantum. We highlight the advantages and disadvantages of using higher-energy states to obtain optimized circuits, compared to default qubit realizations. Our work encourages an investigation of cloud-based quantum circuit optimization techniques using multi-level quantum systems.
Analysis of a Quantum Kernel-Based Classifier Using a Tunable Trapped Ion Noisy Simulator
When: Thursday March 18th | 6PM EDT (Session V32)
Presenter: Keith Kenemer (Aliro Quantum Technologies)
Link: here [Registration required]
In this work, we develop and employ a tunable trapped-ion noisy simulator to analyze the noise-sensitivity of a quantum kernel-based classifier (QKBC) algorithm with respect to various noise metrics specific to existing and near-term trapped-ion hardware. We explore the noise-sensitivity trade-offs by varying several sources of noise during both training and classification. We find that while increasing certain noise levels generically degrades classification performance, training in certain noisy environments can mitigate this effect to some extent. Our results suggest that training in noisy environments and model ensembling could be a promising noise mitigation strategy for QML applications, allowing the model to learn both the characteristics of the data and the noise.
Branching Quantum Convolutional Neural Networks: A Variational Ansatz with Mid-Circuit Measurements
When: Friday March 19 | 8AM EDT (Session X32)
Presenter: Ian MacCormack (University of Chicago)
Link: here [Registration required]
Quantum devices are currently on the cusp of being able to perform tasks beyond the capabilities of classical computers. However, the depth of circuits that can be executed on existing quantum devices is still limited by gate infidelities. In order to take full advantage of near-term quantum computing resources, we must pair them with classical computing resources in intelligent ways. With the branching quantum convolutional neural network (bQCNN), we attempt to do this by introducing a hybrid quantum-classical machine learning ansatz that utilizes mid-circuit measurements to determine which layers of gates to apply. The resulting “branching” structure, where different measurement outcomes lead to different circuit executions, allows us to fit more trainable parameters into a given circuit depth, thereby avoiding additional gate errors incurred by simply using a deeper circuit ansatz while increasing circuit expressibility.
In case you missed it: Geometry of thermal states - thermodynamics of quantum and classical coherence
Presenter: Akira Sone (Los Alamos National Laboratory)
Recording: here [Registration required]
We discuss fluctuation theorems for the conditional stochastic work within the geometric approach, for both quantum and classical dynamics. In the quantum case, the informational contribution due to the back action of the projective measurement plays an important role in formulation meaningful statements of the second law of thermodynamics. In particular, we discuss the contribution of quantum coherence and its corresponding ergotropy, which demonstrates the succinct relationship between work extraction and coherence distillation within geometric stochastic quantum thermodynamics *This work is supported by the U.S. Department of Energy, the Laboratory Directed Research and Development (LDRD) program and the Center for Nonlinear Studies at LANL.
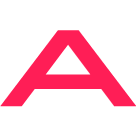