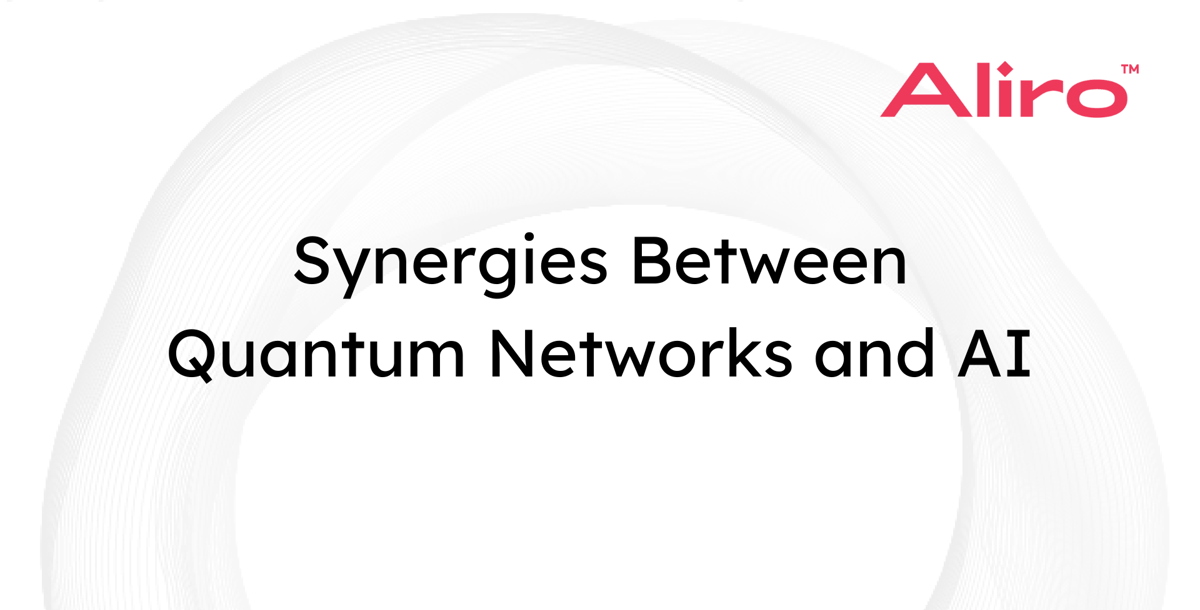
Classical artificial intelligence (AI), quantum AI, quantum computing, and entanglement-based networks each represent new frontiers in technology. Combining classical AI, quantum AI, quantum computing, and entanglement-based networks could lead to new technologies, and may one day revolutionize areas of computing and network communications. In this article, we’ll explore just a couple of those possibilities.
Above: A mindmap of the synergies between quantum technologies and artificial intelligence
Optimizing Network Protocols with Classical AI
The integration of classical AI techniques into the optimization of entanglement-based networks could enhance the performance and reliability of these complex systems. Classical AI reinforcement learning provides a powerful tool to develop more efficient and effective network protocols. Reinforcement learning involves training an AI agent to make a sequence of decisions to achieve a specific goal. The agent learns through trial and error, receiving feedback from the environment to improve performance over time. In entanglement-based networks, reinforcement learning could be used to improve known protocols for entanglement distribution: optimizing how long entanglement is held before it is regenerated, or adaptively managing resources to maximize network performance metrics, such as fidelity. AI could also be used to create and optimize protocols for very specialized cases, such as when two noise channels exhibit different noise characteristics.
AI and ML Privacy in Entanglement-based Systems
In some instances, it is possible to infer certain types of data used to train a machine learning model, posing a significant privacy concern. This issue arises because machine learning models, during their training process, learn and internalize the statistical patterns and relationships present in the training data. Although the primary goal is to generalize from this data for making accurate predictions on new, unseen data, the model's parameters can inadvertently retain information specific to the training data. An attacker with access to the model could use various techniques to extract this sensitive information. Entanglement-based networking technologies offer advanced privacy capabilities that are critical for secure data transmission and computation and could be used to prevent the privacy issue described above. Entanglement-based quantum networks provide information-theoretic security, ensuring the highest degree of data protection. Additionally, using entanglement-based quantum networks enables distributed and blind quantum computing and the creation of quantum client-server architectures that allow for secure execution of algorithms, without revealing any information regarding the data or the algorithm being used on the data.
The fusion of AI and machine learning with entanglement-based technologies has many possible applications. These are very powerful standalone technologies, but they can also be used together synergistically. As these technologies continue to evolve and integrate, they promise to reshape the landscape of computing, communication, and data security, driving innovation and new frontiers in technology.
For more about the synergies between quantum technologies and AI, please see our on-demand webinar, Quantum Networks and AI: Transformative Technologies Unleashed.
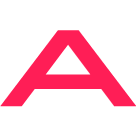